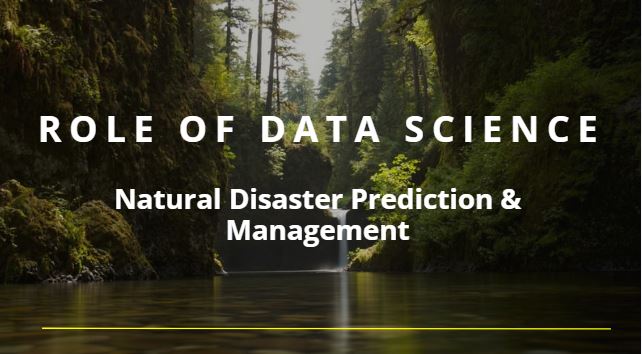
Natural disasters, such as earthquakes, floods, and hurricanes, cause significant disruption to communities and economies. Accurate prediction and efficient management of these disasters can save lives, minimize damage, and improve response efforts. This is where data science has begun to play a critical role, offering innovative tools and models to forecast natural events and optimize disaster response strategies.
In this blog, we will explore how data science is transforming natural disaster prediction and management, from early warning systems to real-time response optimization.
1. The Importance of Early Prediction
Early detection of natural disasters can significantly reduce their impact. Traditionally, scientists relied on historical data and basic models to predict events like hurricanes or floods. However, the accuracy of these predictions was often limited. With the advent of data science, the process has become far more sophisticated. Modern machine learning algorithms can analyze vast datasets, including meteorological patterns, seismic activity, and satellite imagery, to forecast natural disasters with greater precision.
For instance, data-driven models can predict the path and intensity of hurricanes days in advance, giving authorities ample time to organize evacuation plans and prepare emergency responses. These models not only reduce the time lag between detection and action but also improve the accuracy of forecasts, preventing false alarms or missed warnings.
2. Machine Learning in Earthquake Prediction
While earthquakes are notoriously difficult to predict, machine learning is making strides in this area. By analyzing seismic waves, soil data, and tectonic plate movements, machine learning models can identify patterns that may precede earthquakes. These algorithms are trained on large datasets of previous seismic activities and constantly learn to improve their accuracy.
For example, researchers at Stanford University have developed an AI model capable of detecting minor tremors, often precursors to larger earthquakes, offering a potential early warning system. Although the science of earthquake prediction is still evolving, data science tools are enhancing our ability to recognize early signals and mitigate risks.
3. Flood Prediction and Management Using Big Data
Floods are among the most common and devastating natural disasters worldwide. Data science plays a critical role in predicting flood risks by leveraging big data analytics. Governments and disaster management agencies use models that incorporate weather data, rainfall patterns, river flow rates, and land topology to predict the likelihood of flooding in specific regions.
For example, Google partnered with the Indian government to create an AI-powered flood forecasting system that provides real-time warnings to at-risk communities. By processing data from satellite imagery, weather forecasts, and hydrological models, this system can predict flood occurrences up to two days in advance. This early warning allows authorities to evacuate vulnerable populations and take preventive measures like sandbagging and diverting water flow.
4. Hurricane Forecasting with Data Science
Hurricanes are another area where data science is making a significant impact. Data scientists use a combination of atmospheric data, ocean temperature readings, wind patterns, and historical hurricane data to create models that predict the formation, strength, and trajectory of hurricanes.
For example, the National Oceanic and Atmospheric Administration (NOAA) uses machine learning models to track hurricanes and issue real-time updates. These models analyze live satellite data to identify trends in wind speeds, air pressure, and oceanic conditions, allowing meteorologists to forecast the hurricane’s path with increasing accuracy. As a result, communities in the hurricane’s path can receive earlier and more reliable warnings, improving evacuation efforts and reducing casualties.
5. Real-Time Data for Disaster Response
Beyond prediction, data science plays an essential role in disaster management by enhancing response efforts. When natural disasters strike, timely and accurate information is critical for effective decision-making. Data-driven platforms can provide real-time updates on the ground situation, such as which areas are hardest hit and where resources are most needed.
For example, during the 2010 Haiti earthquake, a crisis-mapping tool called Ushahidi was used to crowdsource information from locals about where help was most urgently required. This data was fed into a real-time map that humanitarian agencies used to direct rescue operations. Similarly, drone technology and satellite imagery, coupled with data science models, help authorities assess the extent of damage and deploy resources efficiently in post-disaster recovery efforts.
6. Challenges and Limitations
Despite the advances, predicting natural disasters using data science is not without challenges. Data quality is a major concern. Accurate predictions require reliable, high-resolution data, which is not always available, especially in developing countries. Moreover, some natural phenomena, like earthquakes, remain highly unpredictable due to the complexity of underlying geological processes.
Additionally, ethical considerations around data usage must be addressed. Disaster prediction models often rely on personal and geographical data, raising concerns about privacy and data protection. Therefore, it is crucial for organizations to balance the need for accurate disaster predictions with the ethical use of data.
7. The Future of Data Science in Disaster Management
The future of natural disaster prediction lies in the continued integration of advanced technologies like artificial intelligence (AI), deep learning, and cloud computing. With access to even larger datasets and more powerful computing tools, data science models will only become more accurate and reliable over time.
Innovations such as real-time data streaming, IoT (Internet of Things) sensors, and drone-based data collection are set to revolutionize disaster response by providing instant updates and enabling faster decision-making. Furthermore, partnerships between governments, tech companies, and research institutions will foster the development of more robust predictive models, allowing for more effective disaster preparedness strategies.
Conclusion
Data science has fundamentally changed the way we approach natural disaster prediction and management. By leveraging vast amounts of data, machine learning algorithms, and real-time analytics, we can now forecast disasters more accurately and respond more effectively. As these technologies continue to evolve, the impact of natural disasters will be further minimized, making the world a safer place for everyone.
While challenges remain, the promise of data science in this field is immense, offering hope for more resilient disaster management systems in the years to come.