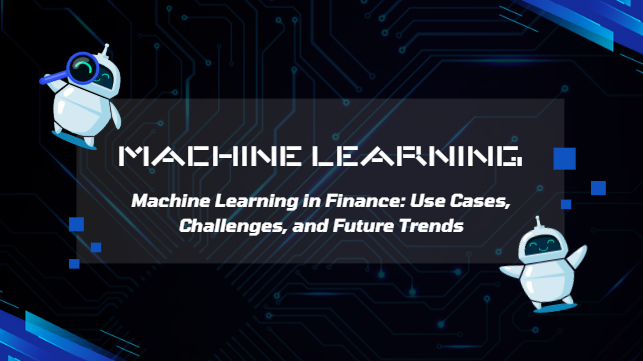
Machine Learning (ML) has become a crucial element of technological advancement, impacting many different industries. In finance, machine learning (ML), a subset of artificial intelligence (AI), is changing the way financial institutions operate. Machine learning has a wide range of innovative applications in banking, from predictive analytics to fraud detection. This blog explores the various applications, challenges, and future developments of machine learning in finance, as well as opportunities to learn more about this fascinating topic.
Use Cases of Machine Learning in Finance
1. Fraud Detection and Prevention
Financial fraud remains a significant issue for banks, credit agencies, and online payment systems. Machine Learning algorithms aid in the detection of fraudulent activities by examining transaction patterns and alerting to abnormalities in real time. For example, ML models can detect suspicious behavior, such as an unusual location for a high-value transaction or abnormal spending habits, reducing financial losses and increasing security.
2. Credit Scoring and Risk Assessment
Small datasets and predetermined criteria are the foundation of traditional credit assessment methods. However, machine learning enables more nuanced risk assessments by considering a diverse set of data points, including alternative credit data, social media activity, and purchase behaviors. This increases accuracy, reduces default rates, and makes it possible for lenders to extend credit to groups that were previously underserved.
3. Algorithmic Trading
With minimal help from humans, algorithmic trading uses machine learning (ML) to assess market trends, close deals, and optimize profitability. These algorithms can process vast amounts of data in milliseconds, discovering patterns and opportunities that human traders may miss. Consequently, trading algorithms driven by machine learning provide a competitive edge in the high-stakes world of financial markets.
4. Personalized Financial Services
Machine learning has made it possible for financial institutions to provide their clients personalized services. Chatbots with AI capabilities, for instance, can provide prompt customer service, and machine learning algorithms can suggest customized investment plans according to each user’s risk tolerance and financial objectives. This level of personalization enhances the user experience and encourages client loyalty.
5. Portfolio Management
Robo-advisors are automated platforms that manage investments and are powered by machine learning. These algorithms optimize portfolios based on market data and individual preferences. Whether you’re an experienced investor or just starting out, machine learning simplifies portfolio management and lowers entry barriers.
Challenges of Implementing Machine Learning in Finance
Even while machine learning has many advantages in the financial industry, there are certain difficulties in putting it into practice:
1. Data Privacy and Security
Since financial organizations deal with sensitive data, security and privacy are important considerations. Ensuring compliance with rules like GDPR and protecting against cyberattacks necessitates strong systems and policies, which might hamper Machine Learning implementation.
2. Bias in Algorithms
The quality of ML models depends on the quality of the data they are trained on. If the training data is skewed, the results will reflect that bias, resulting in unfair choices like discriminating credit approvals. Developing unbiased and transparent models is a continuous problem.
3. Regulatory Compliance
Because of the financial industry’s strict regulations, machine learning models have to abide with intricate regulatory frameworks. Ensuring that ML-driven decisions are explainable and auditable is critical for meeting regulatory criteria, which can bog down innovation.
4. Skill Gap
The need for qualified individuals has increased significantly due to the quick development of AI and ML. Many financial organizations struggle to obtain Machine Learning specialists at all levels, from novice to advanced. Upskilling through AI ML certification programs and Machine Learning courses can help to close this gap.
Future Trends in Machine Learning for Finance
1. Explainable AI (XAI)
Explainable AI is becoming more and more important as regulatory agencies call for increased transparency. Financial institutions will increasingly use ML models to explicitly express their decision-making processes, improving trust and compliance.
2. Integration with Blockchain
The integration of blockchain technology with machine learning has the potential to transform transaction verification and fraud detection. Blockchain’s immutable ledger provides a safe base, while machine learning (ML) adds predictive and analytical capabilities.
3. Decentralized Finance (DeFi)
Machine learning is being used by DeFi platforms to improve risk assessment, maximize yield farming, and increase financial inclusion. The combination of DeFi and machine learning has the potential to disrupt established banking institutions.
4. Improved Customer Experience
AI and ML will enable hyper-personalization in the financial industry of the future, giving clients individualized financial advice, goods, and services. ML algorithms will anticipate user wants, making financial transactions more straightforward.
5. AI-Powered Compliance Tools
For financial organizations, compliance represents a substantial operational expense. Machine Learning will simplify compliance procedures, automate regulatory reporting, and identify potential infractions, lowering costs and increasing efficiency.
Learning Machine Learning for Finance
Many professionals and students are keen to investigate jobs in this industry because of its revolutionary potential. There are numerous AI and machine learning courses and certifications available to meet a variety of skill levels. Whether you’re a beginner looking for a “Machine Learning course near me” or an experienced professional seeking an online AI ML course, there are several tools to help you improve your skills.
Key Learning Pathways:
- AI ML Courses: Extensive courses covering both fundamental ideas and cutting-edge applications.
- Beginner’s Guide to Machine Learning: Foundational courses that provide a solid basis.
- Machine Learning Certification: Courses that provide industry-accepted certifications to verify knowledge.
- Online courses in machine learning: adaptable choices that let you study at your own speed.
- Courses on AI Machine Learning: Expert programs that concentrate on the nexus between AI and ML.
In addition to preparing you for careers in machine learning, upskilling via these channels enables you to participate in the continuous development of the financial sector.
Conclusion
By stimulating innovation, boosting client experiences, and improving decision-making, machine learning is changing the financial environment. Despite challenges such as data privacy, algorithmic bias, and legal hurdles, the future of ML in finance is bright. Staying ahead in this sector necessitates ongoing education, and investing in AI ML certification or an online AI ML course can be a huge step forward.
Professionals with the necessary abilities will have many chances to succeed in the financial industry as the need for ML knowledge increases. Whether you’re new to the discipline or an experienced practitioner, now is the moment to discover Machine Learning’s full potential and make your mark in this changing subject.