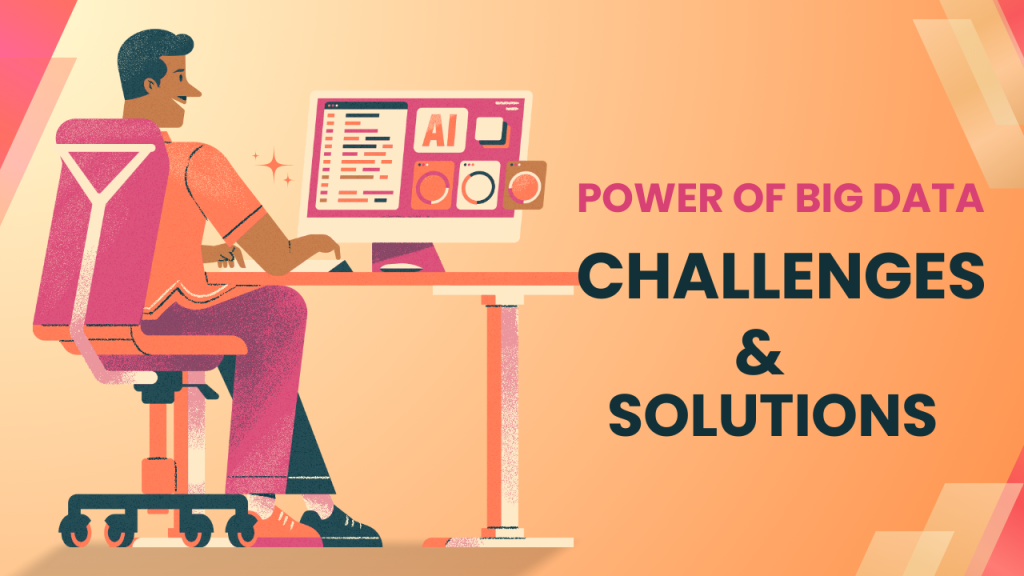
The Big Data Revolution: A Game-Changer for Businesses and Careers
In today’s digital age, we’re surrounded by unprecedented information. From social media posts to online purchases, every click and interaction generates data. This information explosion has given rise to “Big Data.” But what exactly is Big Data, and why should you care?
Big Data refers to the vast volumes of structured and unstructured data that inundate businesses daily. It’s not just about the sheer quantity of data, the velocity at which it’s generated, and the variety of its sources. Understanding Big Data is no longer optional for working professionals and data science students—it’s essential.
As we delve into Big Data, we’ll explore its challenges and solutions and why pursuing data science certifications might be your ticket to a thriving career in this exciting field.
The Three V’s of Big Data: Volume, Velocity, and Variety
Volume: Handling Massive Amounts of Data
Imagine using only a garden hose to fill an Olympic-sized swimming pool with water. That’s the challenge businesses face when dealing with the volume of Big Data. Every day, we create 2.5 quintillion bytes of data. That’s 2.5, followed by 18 zeros!
This massive volume of data presents both opportunities and challenges. On one hand, it provides businesses with a wealth of information to make informed decisions. Conversely, it requires sophisticated tools and techniques to store, process, and analyze effectively.
Velocity: Keeping Up with Real-Time Data
Data is generated astoundingly in the age of social media and IoT devices. Consider this: users send 16 million text messages every minute and watch 4.5 million YouTube videos. The challenge lies in processing this data in real-time to derive actionable insights.
For businesses, the ability to analyze data as it’s being generated can be a game-changer. It allows for immediate response to market trends, customer behavior, and potential issues.
Variety: Managing Diverse Data Types
Big Data comes in all shapes and sizes. From structured data in databases to unstructured data like social media posts, emails, and video content. This variety adds another layer of complexity to data analysis.
Integrating and making sense of these diverse data types requires advanced analytics tools and techniques. It’s like trying to solve a puzzle where the pieces come from different sets—challenging but not impossible with the right approach.
The Big Data Challenge: Why Traditional Methods Fall Short
As data volume, velocity, and variety grow, traditional data processing methods struggle to keep up. Here’s why:
- Scalability Issues: Traditional databases were designed to handle something other than petabytes of data.
- Performance Bottlenecks: Processing massive datasets in real-time requires significant computational power.
- Data Quality Concerns: Data from various sources makes ensuring consistency and accuracy challenging.
- Security and Privacy Risks: As data volumes grow, so do the potential security vulnerabilities.
These challenges underscore the need for innovative solutions and skilled professionals who can navigate the complex landscape of Big Data.
Big Data Solutions: Embracing New Technologies and Approaches
Distributed Computing: Divide and Conquer
One of the most effective solutions for handling big data is distributed computing. This approach involves breaking down enormous datasets into smaller chunks and simultaneously processing them across multiple computers.
- Hadoop: An open-source framework that allows for distributed processing of large data sets across clusters of computers.
- Apache Spark: A fast and general engine for large-scale data processing, capable of handling batch and real-time data.
These technologies enable businesses to process massive amounts of data efficiently, turning a daunting task into a manageable process.
Cloud Computing: Scalable and Flexible Data Storage
Cloud platforms like Amazon Web Services (AWS), Google Cloud, and Microsoft Azure offer scalable storage solutions for Big Data. These platforms allow businesses to:
- Store vast amounts of data without investing in expensive hardware
- Scale resources up or down based on demand
- Access data from anywhere, promoting collaboration and flexibility
For working professionals and data science students, familiarity with cloud computing platforms is becoming increasingly important in the Big Data landscape.
Machine Learning and AI: Extracting Insights from Complex Data
As data sets become more complex, traditional analysis methods fall short. This is where Machine Learning (ML) and Artificial Intelligence (AI) come into play.
ML algorithms can:
- Identify patterns in large datasets
- Make predictions based on historical data
- Improve their performance over time without human intervention
AI technologies like Natural Language Processing (NLP) can analyze unstructured data such as social media posts and customer reviews, providing valuable insights into customer sentiment and behavior.
“Machine Learning is the new electricity.” – Andrew Ng, Co-founder of Coursera.
The Role of Data Science Certifications in Tackling Big Data Challenges
As we’ve seen, Big Data presents numerous challenges requiring specialized skills. This is where data science certifications come into play. These data science certifications equip professionals with the knowledge and skills to navigate the complex world of Big Data.
Why Pursue Data Science Certifications?
- Validation of Skills: Data science certifications will prove your expertise in Big Data technologies and methodologies.
- Career Advancement: With the growing demand for Big Data professionals, certifications can open doors to new opportunities and higher-paying roles.
- Continuous Learning: The field of Big Data is constantly evolving. Data science certifications ensure you stay up-to-date with the latest trends and technologies.
- Networking Opportunities: Many certification programs offer access to communities of like-minded professionals, providing valuable networking opportunities.
Popular Data Science Certifications
- IBM Data Science Professional Certificate: Covers various data science topics, including big data and machine learning.
- Google Professional Data Engineer: Focuses on designing and building data processing systems on Google Cloud Platform.
- Microsoft Certified: Azure Data Scientist Associate: Emphasizes using Azure Machine Learning for implementing and running machine learning workloads.
- Cloudera Certified Associate (CCA) Data Analyst: Demonstrates SQL and query writing proficiency for Apache Hadoop.
These data science certifications enhance your skills and make you more attractive to potential employers in the Big Data field.
Real-World Applications: Big Data in Action
To truly appreciate the power of Big Data and the importance of data science skills, let’s look at some real-world applications:
Healthcare: Predictive Analytics for Better Patient Care
Big Data analytics is revolutionizing healthcare by:
- Predicting disease outbreaks based on social media data and search trends
- Analyzing patient records to identify individuals at high risk of certain conditions
- Optimizing hospital staffing based on predicted patient influx
Finance: Fraud Detection and Risk Management
In the financial sector, Big Data is used for:
- Detecting fraudulent transactions in real-time
- Assessing credit risk more accurately
- Predicting market trends for better investment decisions
Retail: Personalizing Customer Experience
Retailers leverage Big Data to:
- Analyze customer behavior for personalized product recommendations
- Optimize pricing strategies based on demand and competition
- Improve supply chain efficiency through predictive analytics
These examples highlight the transformative power of Big Data across industries, underscoring the value of data science skills in today’s job market.
The Future of Big Data: Trends to Watch
As we look to the future, several trends are shaping the Big Data landscape:
- Edge Computing: Processing data closer to where it’s generated, reducing latency and improving real-time analytics.
- Augmented Analytics: Combining AI and ML to automate data preparation, insight discovery, and sharing.
- Data Privacy and Ethics: With increasing concerns about data privacy, there’s a growing focus on ethical data use and robust security measures.
- Quantum Computing: While still in its early stages, quantum computing promises to revolutionize Big Data processing capabilities.
Conclusion: Big Data Challenge
As we’ve explored, Big Data presents significant challenges and exciting opportunities. From the sheer volume of data to the complexity of analysis, Big Data is not for the faint of heart. However, with the right skills and tools, these challenges can be transformed into powerful insights that drive business success.
For working professionals and data science students, the message is clear: embracing Big Data is no longer optional—it’s essential for career growth and success in today’s data-driven world. Pursuing data science certifications and staying updated with the latest trends and technologies can position you at the forefront of this exciting field.