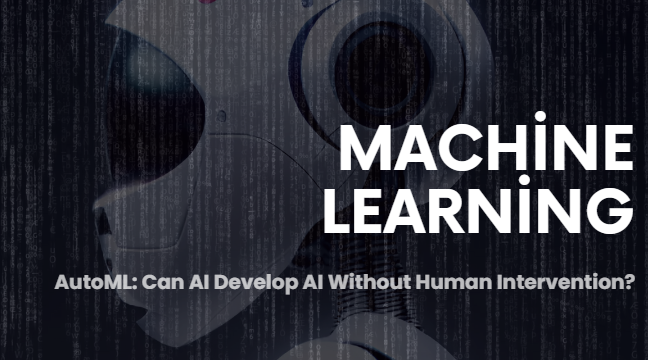
Through process optimization, decision-making improvement, and the automation of complicated operations, artificial intelligence (AI) and machine learning (ML) have completely transformed a number of sectors. One of the most intriguing developments in this field is Automated Machine Learning (AutoML), which seeks to automate the process of creating machine learning models. This poses a fundamental question: Can AI generate AI without human intervention? Let’s discuss the concept of AutoML, its potential, limits, and the role of human expertise in defining its future.
What is AutoML?
The goal of the AI and machine learning field of autoML is to automate the entire process of applying ML models to real-world issues. Data preparation, feature engineering, model selection, hyperparameter tuning, and evaluation are all stages in the traditional ML model development pipeline. These activities necessitate extensive knowledge, making AI ML courses and machine learning training indispensable for professionals. However, AutoML seeks to reduce human participation by expediting these stages through intelligent automation.
How AutoML Works
AutoML systems use a variety of methods to create effective machine learning models without human assistance. These techniques include the following:
- Neural Architecture Search (NAS): NAS enhances the model’s performance and efficiency by automatically locating the optimal deep learning architecture.
- Hyperparameter Optimization: To improve model correctness, AutoML adjusts hyperparameters including learning rates and batch sizes.
- Selection and Feature Engineering: AutoML finds the most pertinent characteristics, simplifying and enhancing predictions.
- Model Selection: Using the provided dataset as a guide, it evaluates many machine learning algorithms and chooses the best model.
- Ensemble Learning: AutoML improves overall predictive performance through the combination of several models.
These developments make AutoML an effective tool that lessens the requirement for substantial human involvement in the creation of AI models.
Benefits of AutoML
1. Democratizing AI
Without needing in-depth understanding of Machine Learning methods, autoML allows non-experts to take advantage of AI capabilities. This democratization enables firms to implement AI technologies without recruiting big teams of data scientists.
2. Speed and Efficiency
AutoML greatly reduces the time needed for experimentation and model development by enabling enterprises to rapidly construct and deploy ML models.
3. Cost Reduction
AutoML reduces the overall cost of AI adoption by reducing the requirement for highly skilled AI personnel, which is particularly advantageous for small organizations who cannot afford dedicated ML teams.
4. Improved Model Performance
In order to choose the best-performing model for a task, AutoML methodically tests various models and hyperparameters.
5. Scalability
AutoML systems are appropriate for businesses with high data requirements since they can grow easily and manage enormous datasets.
Limitations of AutoML
There are some drawbacks to AutoML despite its many benefits. Some of the significant challenges are:
1. Lack of Domain Expertise
In complicated applications, autoML tools might not fully comprehend domain-specific subtleties, which could result in less than ideal model performance. Professionals with machine learning certificates are still necessary to interpret data and refine models.
2. Data Quality Dependence
AutoML systems are very dependent on well-labeled, high-quality data. Poor data quality might produce erroneous models, necessitating human involvement for data cleaning and preprocessing.
3. Limited Customization
AutoML may not be appropriate for highly specialized solutions that call for expertly developed architectures, despite its ability to optimize basic ML models.
4. Computational Cost
Due to their high computational resource requirements, several AutoML techniques—like Neural Architecture Search—are costly to implement.
5. Ethical and Bias Concerns
Existing datasets, which may have biases, are used to train autoML models. Without human intervention, these biases can be strengthened, resulting in unfair or discriminating outcomes.
The Role of Humans in AutoML
Even though AutoML can automate tasks, human intervention is still necessary. Human expertise is critical in numerous fields.
- Identifying the Issue: For ML model creation to be successful, it is essential to comprehend business requirements and formulate an appropriate problem statement.
- Data Preparation: Accurate model training requires high-quality, pertinent data.
- Model Interpretation: Although AI may produce very effective models, human specialists are still required to evaluate the outcomes and make sure they support corporate goals.
- Ethical AI Implementation: Human supervision is necessary to address biases and guarantee responsible AI deployment.
- Continuous Learning and Improvement: Experts certified in online machine learning courses are able to improve AutoML models in response to shifting business needs.
The Future of AutoML
AutoML is positioned to play a crucial role in the development of AI and ML, increasing the efficiency and accessibility of AI. However, comprehensive AI automation without human intervention remains a distant reality. Future advancements will probably focus on:
- Better Explainability: To make AI models easier to understand, AutoML decision-making should be more transparent.
- Improved Domain Knowledge Integration: Creating AutoML systems that use domain knowledge to produce predictions that are more pertinent and accurate.
- Ethical AI Development: Reducing bias in AutoML-generated models by implementing fairness-aware algorithms.
- More Efficient Computation: Lowering computational expenses to increase AutoML’s usability for a larger group of people.
Conclusion
In order to automate the creation of AI models, lessen reliance on human knowledge, and increase accessibility to AI, autoML is an important step. However, AI cannot fully grow without human intervention. Human knowledge remains essential for defining problems, assuring data quality, evaluating outcomes, and dealing with ethical concerns.
Enrolling in AI and Machine Learning courses or acquiring a machine learning certification can give professionals the abilities they need to become experts in AutoML and AI development. With the correct mix of automation and human expertise, the future of AI and ML is bright and transformational.